AI-Based Video Analysis for Driver Fatigue Detection: A Literature Review on Underlying Datasets, Labelling, and Alertness Level Classification
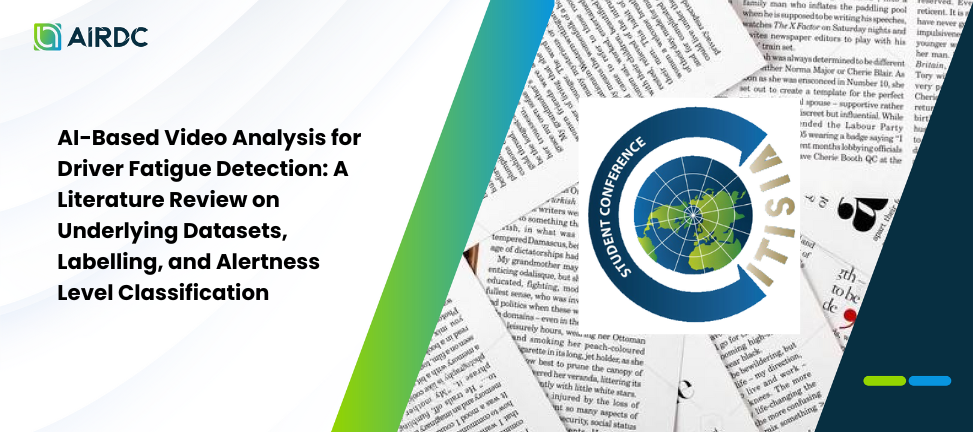
Reduced alertness because of fatigue or drowsiness accounts for a major cause of road accidents globally. To minimize the likelihood of alertness reduction-related crashes, a video-based detection emerges as a non-intrusive method and can offer high accuracy for the countermeasure development. Many Artificial Intelligence (AI) techniques have been developed to aid in video analysis for fatigue detection. However, the issues in AI-based video analysis for fatigue countermeasure development are rarely discussed. This paper reviews current video-based countermeasure development in the literature and highlights some reliability issues of AI-based fatigue countermeasure development in terms of underlying dataset, labelling method, and alertness level classification. The result serves as insights and consideration to academics and industrialists in developing a high reliable AI-based video analysis for driver fatigue countermeasure.
Authors:
Dedy Ariansyah, Reza Rahutomo, Gregorius Natanael Elwirehardja, Faisal Asadi, and Bens Pardamean
International Conference on Innovative Technologies in Intelligent Systems and Industrial Applications, CITISIA 2022