Systematic Literature Review of Transformer Model Implementations in Detecting Depression
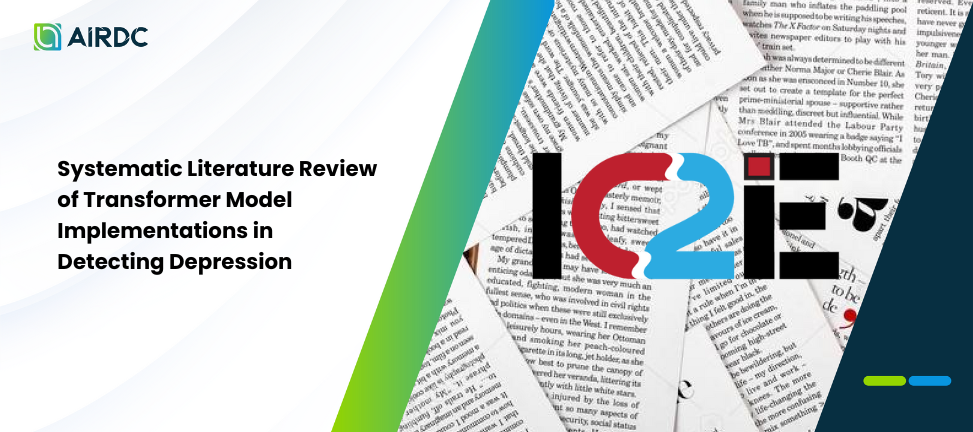
To enable early detection of human depression, deep learning models such as transformers have been a feasible approach. This systematic literature review examines the application of transformer models in detecting depression across text, audio, and video modalities. The study finds that transformer architectures, particularly BERT for text data, effectively capture contextual information and linguistic patterns related to depression. Hybrid approaches combining transformer models with other architectures are commonly used for audio and video data. Important features include eye gaze, head pose, facial muscle movements, audio features such as MFCC and Log-mel Spectrogram, and text embeddings. Performance comparisons indicate that text-based data consistently yields the best results, followed by audio and video modalities using Transformer model. Combining multiple modalities improves performance, with the combination of audio, video, and text achieving the most accurate predictions. Unimodal approaches also show promise, with text data outperforming audio and video data. The review identifies challenges in this research, such as imbalanced datasets, limited availability of comprehensive samples, and difficulties in interpreting visual cues.
Authors:
Kenjovan Nanggala, Bens Pardamean, and Gregorius Natanael Elwirehardja
6th International Conference on Computer and Informatics Engineering, IC2IE 2023