Machine Learning Approach for Single Nucleotide Polymorphism Selection in Genetic Testing Results
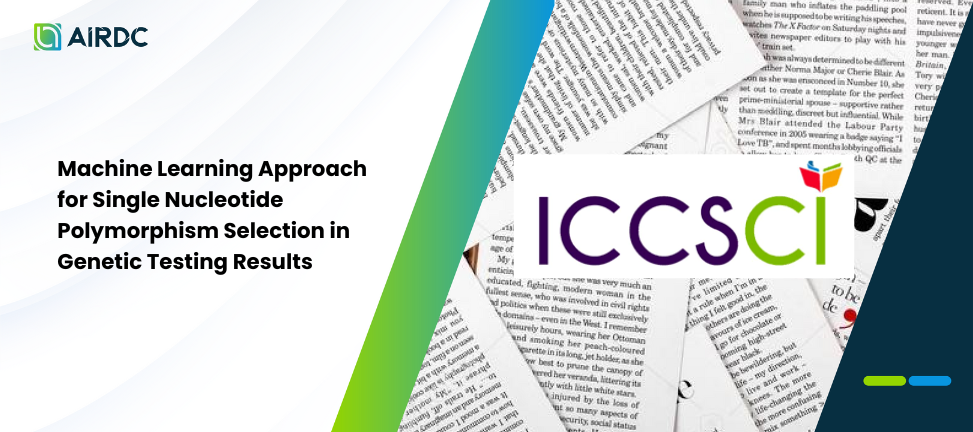
Lactose intolerance is a type of digestive problem that may threaten the population because milk and dairy products compose of nutrients that are essential for human body. Genetic tests possess a great potential to detect lactose intolerance as it can be used in children and even infants. However, a new approach to analyze the genetic test results is needed to elucidate the Single Nucleotide Polymorphisms (SNPs) that are related to lactose intolerance. In this work, we utilized the machine learning based feature selection to select the SNPs associated with lactose tolerance trait from genotyping samples of direct-to-customer (DTCG genetic tests, obtained from the public database. Recursive Feature Elimination (RFE) with XGBoost model was used to perform feature selection. We also compared three different models, such as XGBoost, support vector machine (SVM), and random forest (RF) for training the selected features. Our findings revealed that 20 SNPs (out of 3501) were chosen, with rs4394668 as the most important variables (F-score 0.009). Furthermore, when compared to the RF and SVM models, the XGBoost model had the highest accuracy (0.87). Further studies should be undertaken to elucidate how the selected SNPs may lead to the lactose intolerance trait.
Authors:
Joko Pebrianto Trinugroho, Alam Ahmad Hidayat, Mahmud Isnan, and Bens Pardamean
8th International Conference on Computer Science and Computational Intelligence, ICCSCI 2023