SNP Distributed Representation Using Entity Embedding
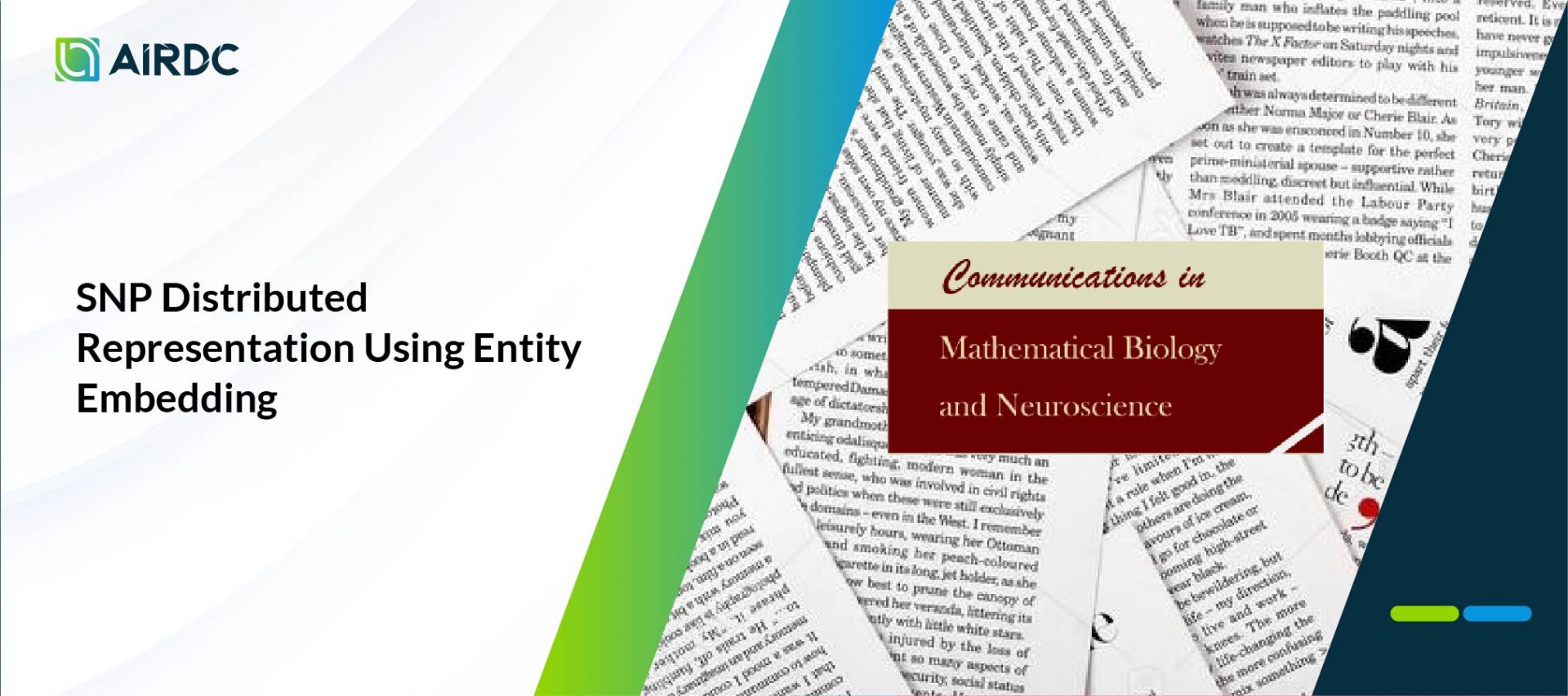
A single Nucleotide Polymorphism (SNP) array is the largest variation of genetic information to detect specific traits in organisms. SNP is located in a specific locus of DNA sequences. To the day this study was conducted, the representation of SNPs for machine learning models is still questionable. Based on the previous works, we proposed a comparative study of distributed representation methods against SNPs data. This study used 1,232 SNPs from the genomic data of 687 Indonesian rice samples collected from four distinct rice fields. The SNP data used was converted into an encoded format. Entity embedding (Embedder) and several comparative models, i.e., Node2Vec, Struc2Vec, and LINE, were chosen to predict the rice yield of the SNP data. The entity embedding using Embedder outperformed the comparative methods used in this study, namely Node2Vec, Struc2Vec, and LINE with the best R2 and MSE scores of 0.9368 and 0.2425 respectively.
Communications in Mathematical Biology and Neuroscience
Francisco Ferano, Jonathan Christian Setyono, Ardivo Virsa Siswanto, Nicholas Dominic, Bens Pardamean