Evaluating Self-Supervised Pre-Trained Vision Transformer on Imbalanced Data for Lung Disease Classification
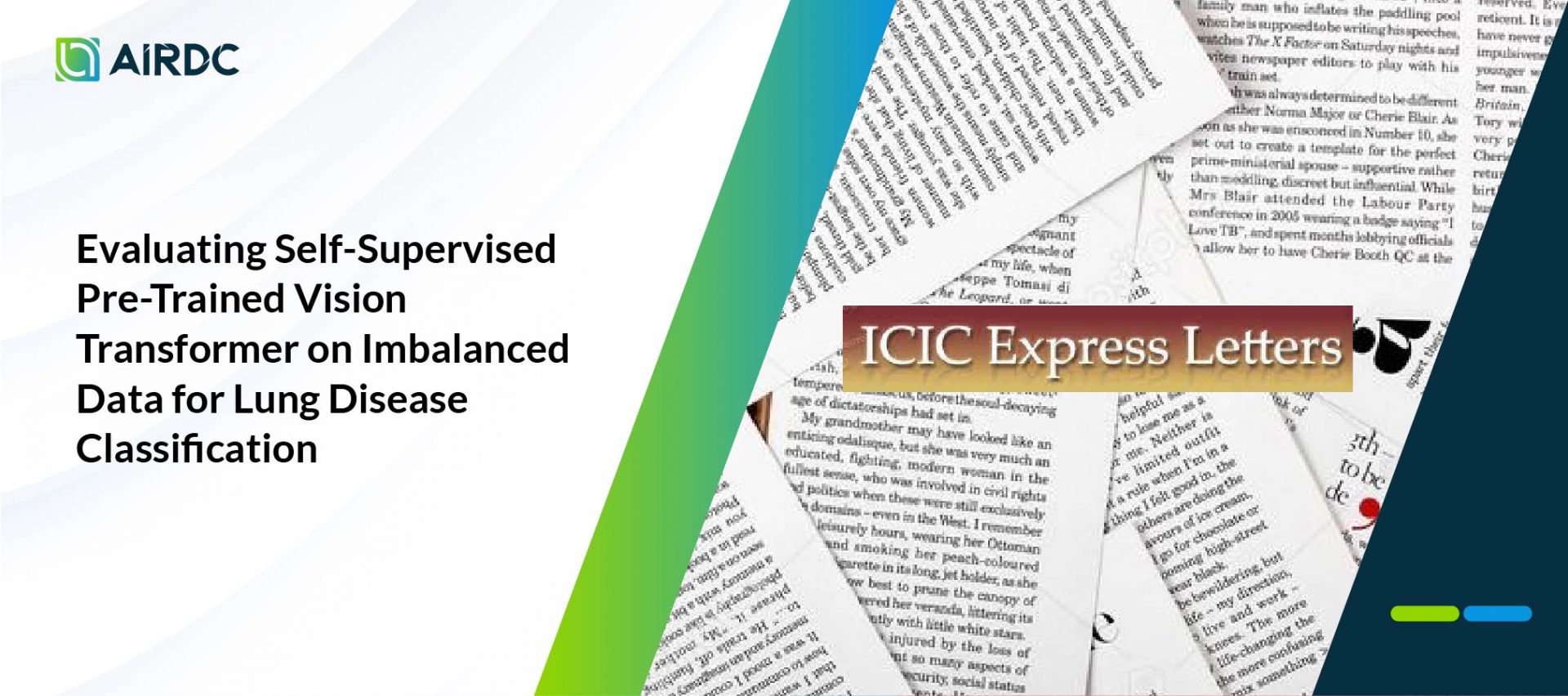
Lung disease has been known as one of the most prevalent medical disorders globally and a leading cause of death and disability. Pneumonia, one of the most common lung diseases, accounts for over 2.4 million deaths annually, and COVID-19 has further increased deaths from pneumonia globally. Chest X-Ray (CXR) has been proven as the most prominent screening method, and deep learning techniques have been widely used for computer-aided diagnosis (CAD). This paper aims to evaluate the performance of Vision Transformer (ViT), self-supervised learning (SSL) techniques, and pre-trained convolutional neural network (CNN) models in classifying four lung conditions from publicly available dataset containing more than 20,000 CXR images. The results showed that DINO ViT-S16 performs the best with precision/recall/F1-score of 95.61%/95.75%/95.67% for the imbalanced dataset, 94.16%/94.56%/94.35% for the augmented dataset, and 93.99%/94.05%/93.86% for the undersampled dataset. The lung regions from the CXR image were correctly highlighted by the model which contributed towards the correct classification. The proposed model also offered higher performance than other previously reported approaches and provides the opportunity for an efficient evaluation with an accuracy acceptable in the medical area.
ICIC Express Letters
Elvan Selvano, Aedentrisa Yasmanda Paulindino, Gregorius Natanael Elwirehardja, Bens Pardamean