A Hybrid CNN-SVM Approach for Malaria in Blood Cell Detection
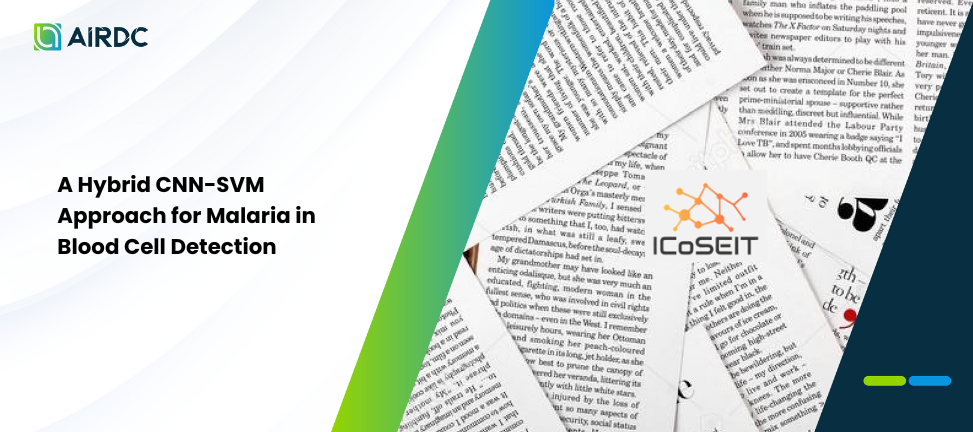
There is still a severe malaria problem worldwide, particularly in regions with limited access to diagnostic tools. It is crucial to develop a system for detecting malaria in blood cells. This paper presents a hybrid Convolutional Neural Network (CNN), and as a classifier, we use a Support Vector Machine (SVM) framework for the automated detection of malaria parasites in blood cell images. The proposed system leverages the strengths of CNNs in feature extraction and representation learning from images, combined with the discriminative power of SVMs for classification. Initially, CNN extracts intricate features from blood cell images, capturing essential patterns indicative of malaria infection. Subsequently, the extracted features are used to train an SVM classifier, enabling accurate discrimination between parasitized and uninfected blood cells. Experimental dataset evaluations were obtained from the Lister Hill National Center for Biomedical Communications website of the National Library of Medicine. The proposed model achieves a better f1-score, outperforming individual CNN or SVM models, around 0.015 compared to individual CNN models and 0.27 compared to individual SVM models. This hybrid CNN-SVM methodology offers a promising solution for accurately and efficiently detecting malaria parasites in blood cell images.
Authors:
Andrea Stevens Karnyoto, Mahmud Isnan, Bens Pardamean
2024 2nd International Conference on Software Engineering and Information Technology (ICoSEIT)