Performance Evaluation of Coffee Bean Binary Classification Through Deep Learning Techniques
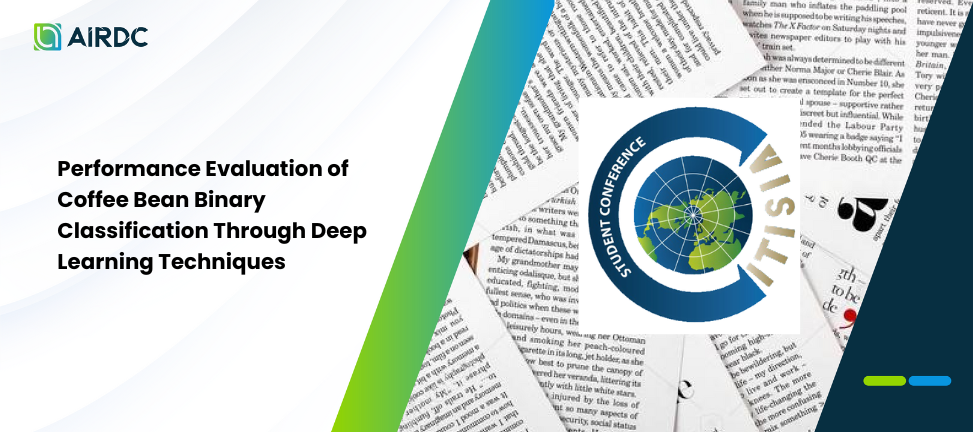
Coffee beans are one of the high-value commodities in Indonesia, but the sorting method for the quality of coffee beans still uses visual methods and sieves with mechanical machines. This study aims to provide an alternative to classifying coffee beans using Deep Learning algorithms, such as the ResNet-18 and MobileNetV2. The dataset used is USK-COFFEE which consists of two classes, the normal coffee bean class, and the defect coffee bean class. The models were trained using the balanced and imbalanced datasets, meaning that two scenarios were performed. From the results of this study, the accuracy value for the ResNet-18 model was 91.50% when trained on the balanced dataset and 90.87% on the imbalanced dataset. It outperformed the MobileNetV2 architecture, which only achieved 0.8125 and 0.8112 accuracy scores when trained on the balanced and imbalanced datasets, respectively.
Authors:
Fajrul Islamy, Kahlil Muchtar, Fitri Arnia, Rahmad Dawood, Alifya Febriana, Gregorius Natanael Elwirehardja, and Bens Pardamean
International Conference on Innovative Technologies in Intelligent Systems and Industrial Applications, CITISIA 2022