Long Short-Term Memory-based Models for Sleep Quality Prediction from Wearable Device Time Series Data
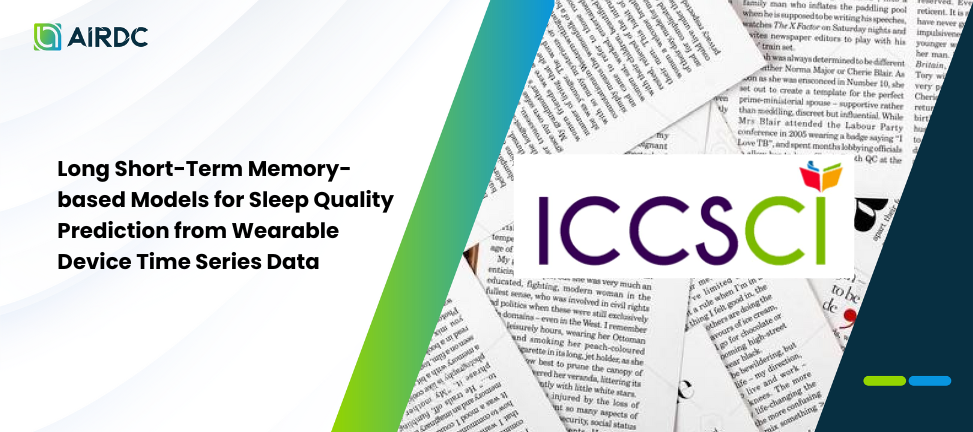
Several studies suggest that sleep quality is considered to be associated with physical activities. Moreover, deep sleep time can be used to determine the sleep quality of an individual. In this work, we aim to find the association between physical activities and deep sleep time by modeling the time series data such as heart rate and a number of steps captured from a commercial wearable device. Our previous study demonstrates that deep learning-based time series modeling is well suited for our problem since the temporal patterns in the two physical parameters need to be captured to obtain more accurate results. We first preprocess our series data to have a time-step size of 10 minutes. To improve our previous effort in this modeling, we compare four different variants of Long Short Term Memory (LSTM)-based models, ranging from single input to dual input models. Our result shows that the simple stacked LSTM model performs better for our data because the remaining models suffer from overfitting due to a larger number of the trained parameters.
Authors:
Alam Ahmad Hidayat, Arif Budiarto, and Bens Pardamean
8th International Conference on Computer Science and Computational Intelligence, ICCSCI 2023