Count-Data Mixed Models of Topical Tweets: A Case of Indonesia Flood Events
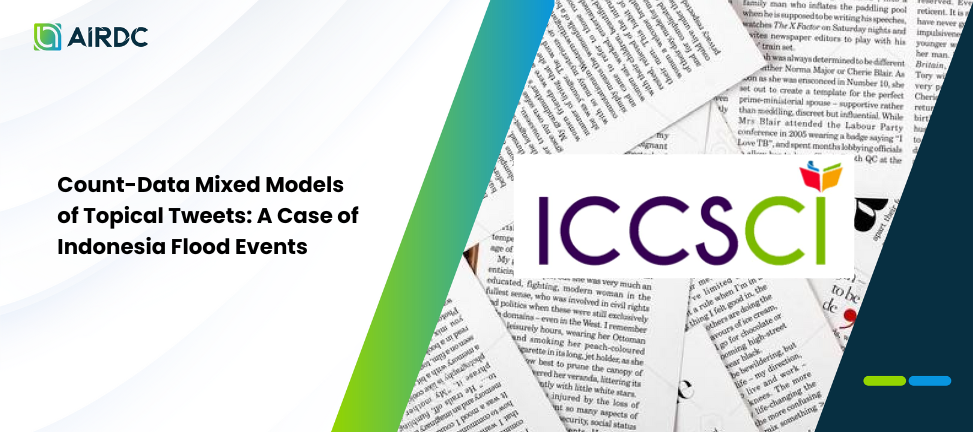
A topic-level variability in modeling twitter data can potentially generate more comprehensive conclusions about the public perception during critical times for improving natural disaster mitigation and surveillance efforts. We employ generalized linear mixed models to demonstrate the variability in Indonesian topic-specific tweet count data during the flood events in February 2021 using the glmmTMB library in R. The data are assumed to be generated from two different exponential distributions: Poisson and Negative Binomial. We implement random effects by allowing random intercepts and random slopes to vary across topics randomly in the two first models. Furthermore, the dispersion and zero-inflation problems are also addressed in the final model. Using Akaike Information Criteria scoring, we obtain that a Negative binomial-based model with random zero-inflation intercepts is favored by the data. The chosen model formulation and the estimated parameters may be useful to forecast topic-specific trends in Indonesian flood-related Twitter data.
Authors:
Alam Ahmad Hidayat and Bens Pardamean
8th International Conference on Computer Science and Computational Intelligence, ICCSCI 2023