Energy-efficient deep learning model for fruit freshness detection
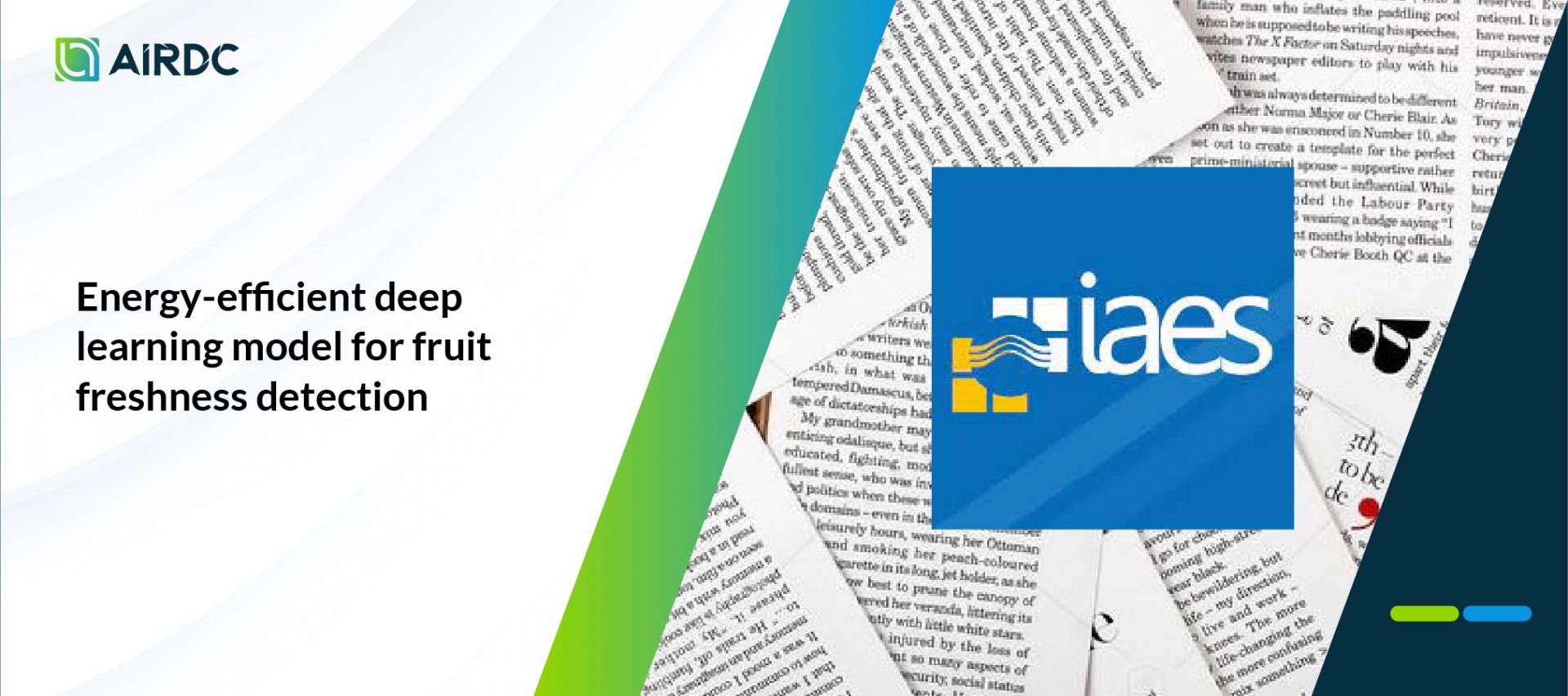
Fruits are usually used as complementary foods because they contain good nutrients such as protein and vitamins. In addition to having good content, it turns out that there are potentially harmful microorganisms contained in fruits caused by decay. Currently, many artificial intelligence (AI) techniques have been proposed in research related to fruit freshness. Deep learning is one of its most prominent types in similar studies. As deep learning typically requires a lot of computation power, it usually consumes a lot of electricity. This is an important concern, especially for agribusiness companies that require AI implementations. Based on these problems, we propose to build a convolutional neural network (CNN) model consisting of six layers to detect fruit freshness and save energy. The CNN model we built uses electrical power ranging from 55 to 73 Watts during the training process and 20 to 27 Watts during the testing process. For accuracy, the result is 98.64%. However, compared to previous studies with the MobileNetV2 model, our model only excels in several aspects, such as recall in fresh banana and fresh oranges, recall and F1-score in Rotten Banana.
IAES International Journal of Artificial Intelligence
Febrian Valentino, Tjeng Wawan Cenggoro, Gregorius Natanael Elwirehardja, Bens Pardamean