Machine Learning Implementations in Childhood Stunting Research: A Systematic Literature Review
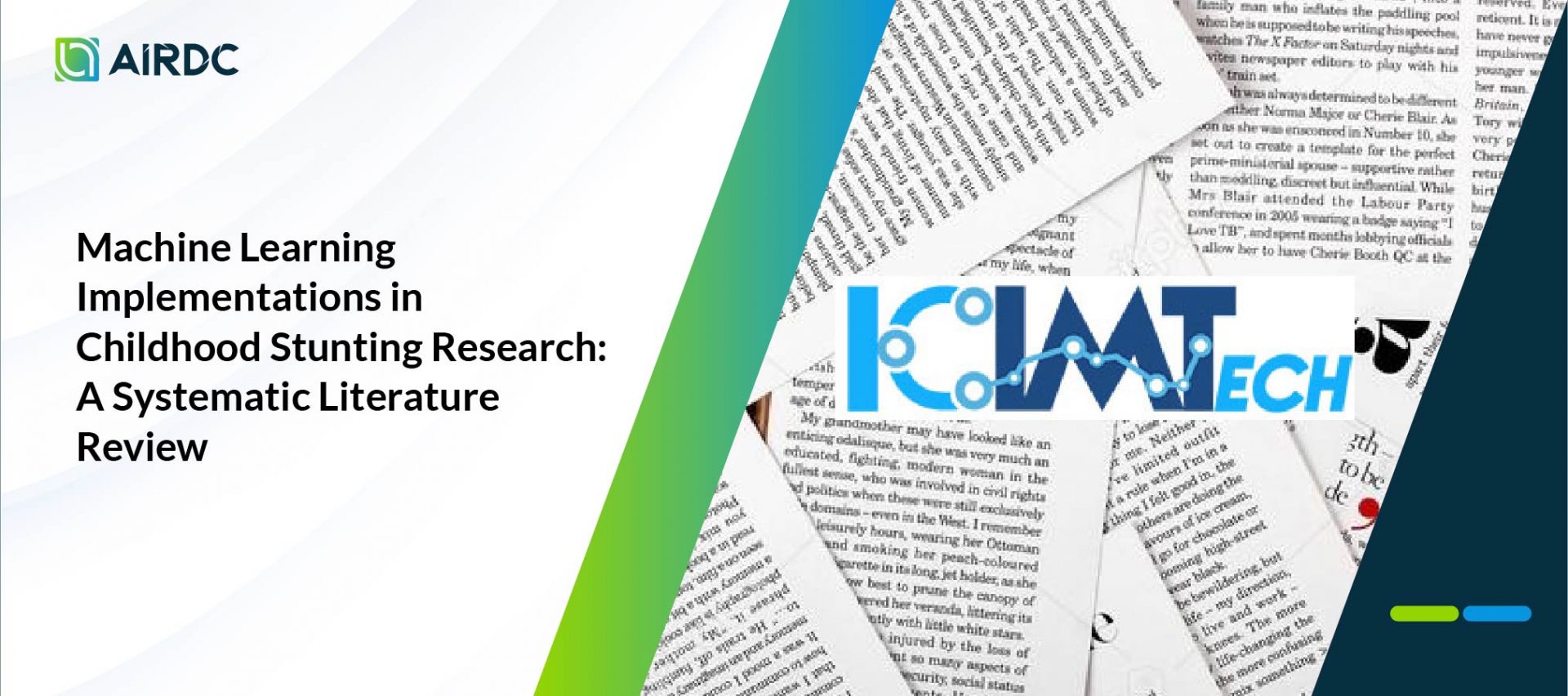
Childhood stunting is a condition anticipated to affect the growth potential of children under the age of five. With numerous stunting researches that have been conducted, stunting datasets are now widely available to facilitate stunting research. This provides an opportunity to implement machine learning (ML) principles to produce a broader insight or a novel technique in stunting prediction. A systematic literature review is necessary to discover the landscape of machine learning implementation in the application domain as a preliminary study for creating an effective research roadmap. This paper presents a systematic literature review (SLR) of 22 curated manuscripts that focuses on identifying the ML models applied in stunting research, as well as the datasets used in such studies that were published during 2017–2022. The SLR process found that ML principles have been applied in stunting research since 2017, and the diversity of ML implementation has become more varied in 2021–2022. In terms of ML models, XGBoost and Random Forest are recognized as the two most utilized models, and stunting prediction is the most common ML implementation. The majority of stunting research utilizing ML has been conducted in Indonesia. Although national survey data has been the most commonly utilized dataset in stunting research, researchers in Indonesia have shown a preference for utilizing data from regional or independent surveys. This study will be followed by developing a classifier model for stunted children using XGBoost and Random Forest algorithms. The model will be trained on a dataset generated from StuntingDB.
ICIMTech
Reza Rahutomo, Gregorius Natanael Elwirehardja, Mahmud Isnan, Faisal Asadi, Bens Pardamean