Counting People Inside A Region-Of-Interest In CCTV Footage With Deep Learning
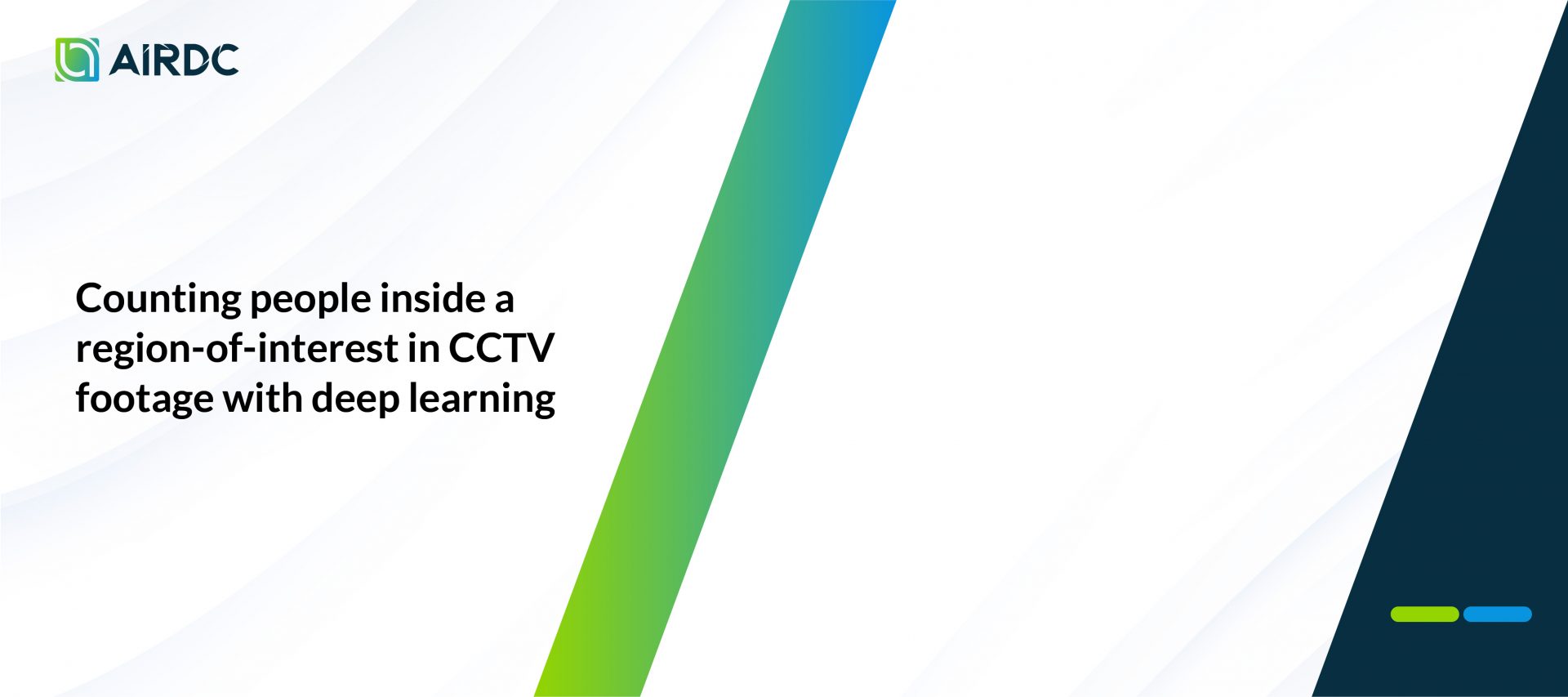
Covid-19, a global challenge in recent years, has changed various aspects of life in Indonesia and around the world. In an effort to control the spread of this virus, effective measures are needed to limit crowds in various regions. This is where the people counting model becomes important. In recent years, technological developments have allowed the development of more sophisticated people counting models. However, current models are still limited in their ability to count the people detected in the overall CCTV footage. However, in the context of Covid-19, we often only need to count the number of people in certain areas that are the focus of the Region of Interest (RoI) in the recording. One simple approach one might think of is to cover areas outside of the RoI to ignore those outside of those areas. However, this approach can reduce model performance and is less practical to implement. Therefore, a smarter and more efficient solution is needed to calculate the number of people in RoI without sacrificing model performance. In the context of Covid-19, where distance and crowd restrictions are very important, an accurate and effective people counting model is urgently needed. By combining technological developments and more sophisticated approaches, it is hoped that we can develop a people counting model that is able to identify the number of people in RoI with a high degree of accuracy. This will help control the spread of the virus, ensure compliance with social distancing rules, and make a positive contribution to the fight against Covid-19, both in Indonesia and around the world.
The BSDRC research team has created a web application system to monitor violations of the maximum capacity of people indoors using computer vision technology based on artificial intelligence (Artificial Intelligence / AI). This system uses an AI model in the form of CSRNet which is equipped with a gap regularizer to calculate the number of people in the Region-of-Interest (RoI). So far, three CSRNet models have been used in the system, each of which has been trained using CCTV recording data from different places with different RoI. This model will count the number of people and mark their location as long as they are in the RoI. If the number of people counted exceeds the maximum capacity of the room where the CCTV is placed, a warning will be displayed on the system. The heatmap image below shows the detection results displayed along with the number of people detected.
It is hoped that with such a system, building managers such as offices or campuses can maintain compliance with the maximum room capacity more effectively and efficiently without worrying about outdoor areas being caught by CCTV, such as rooms with glass partitions. Thus, the risk of transmission of COVID-19 can be reduced again.