Offline Signature Verification Using Transfer Learning and Data Augmentation on Imbalanced Dataset
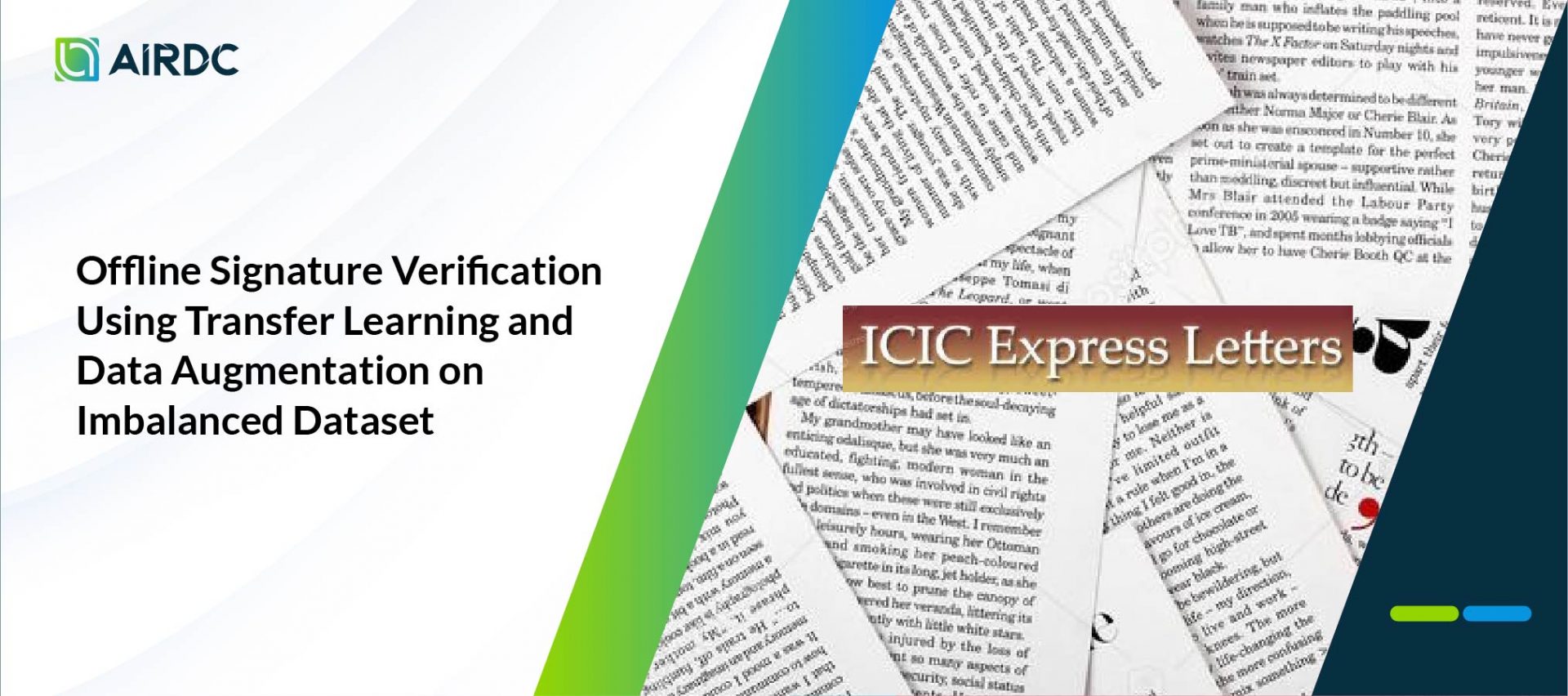
Handwritten signature verification has attracted a lot of attention in recent years for authentication purposes and yet, it is still prone to mistakes of human error. Thus, with the development of deep learning, many methods of signature forgery classification were proposed in previous studies. However, not many studies have addressed the problem of imbalanced datasets which have been known to affect the models’ performance. Therefore, this paper aims to solve the problem of imbalanced dataset by using transfer learning and multiple data augmentations. Transfer learning provides efficiency by using a pre-trained model for enhanced performance. Additionally, data augmentation applies transformations to enlarging datasets. Hence, four approaches were evaluated in this study. The first three approaches used a custom Convolutional Neural Network (CNN) trained on different datasets: 1) an imbalanced dataset, 2) a balanced dataset, and 3) an augmented balanced dataset. Meanwhile, the fourth approach used a pre-trained VGG16 via transfer learning trained on an augmented balanced dataset. The result of our study shows that among our proposed approaches, the transfer learning of the VGG16 model trained on an augmented balanced dataset outperformed the replicated baseline models with 86.7% accuracy.
ICIC Express Letters
Aeron Mitchell, Evan Edbert, Gregorius Natanael Elwirehardja, Bens Pardamean