Sequence to Sequence Deep Learning Architecture for Forecasting Temperature and Humidity inside Closed Space
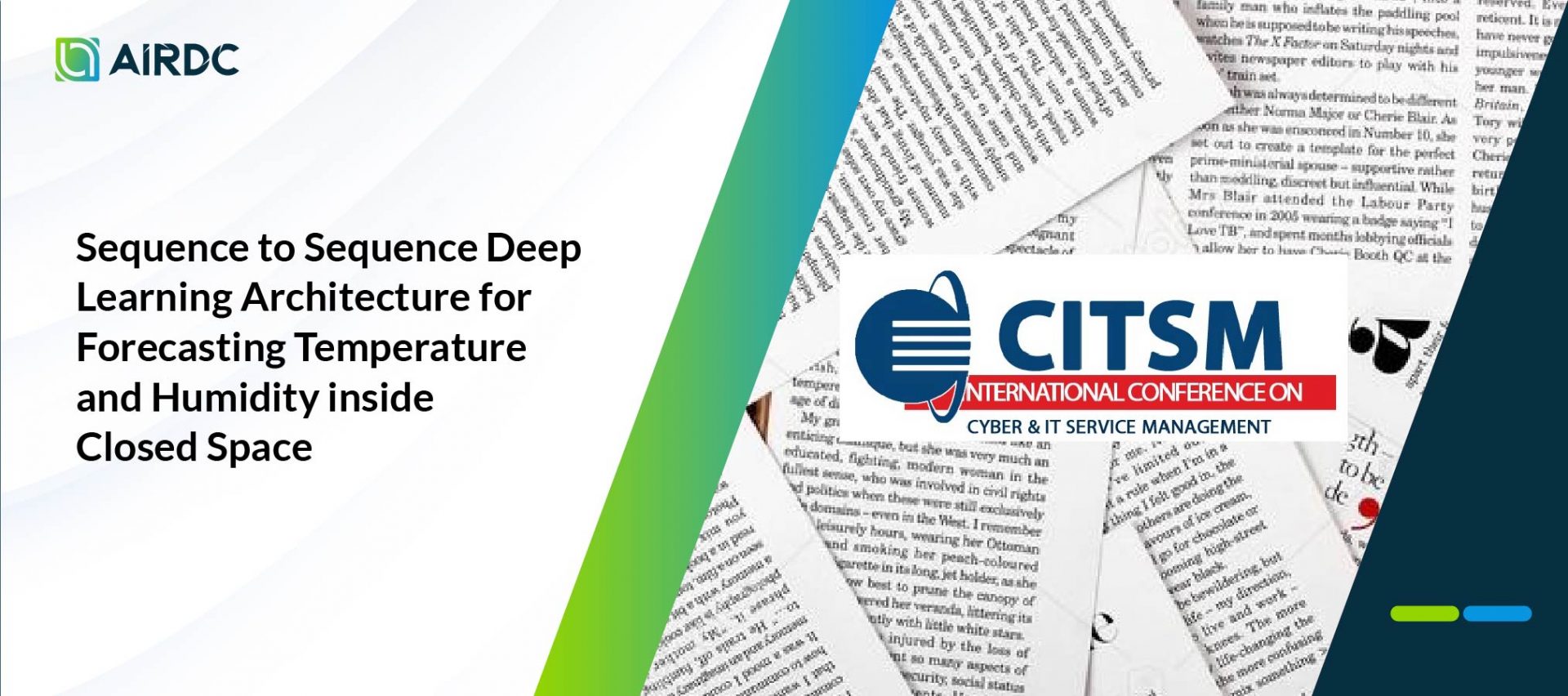
Solar Dryer Dome (SDD), an agricultural facility for drying and preserving agricultural products, needs a smart ability to predict the future indoor climate accurately, including indoor temperature and indoor humidity, in order to optimize electricity usage. To overcome these challenges, deep learning has been a widely adopted method. This research aims to forecast the future indoor climate using time series data by implementing a sequence-to-sequence (seq2seq) architecture, which is mostly used in Natural Language Processing (NLP) tasks. The two proposed seq2seq models, Long Short-Term Memory (LSTM) seq2seq and Gated Recurrent Unit (GRU) seq2seq, have proven to be superior to the adapted LSTM and GRU. The results show that the seq2seq GRU model outperforms the adapted GRU baseline model by an average difference of 0.03013 in MAE and the seq2seq LSTM model outperforms the adapted LSTM baseline model by an average difference of 0.00941 in MAE. To the best of our knowledge, this is the first implementation of seq2seq models for indoor climate forecasting on the Room Climate dataset.
International Conference on Cyber and IT Service Management
Karli Eka Setiawan, Gregorius Natanael Elwirehardja, Bens Pardamean