Evolving Hybrid Cascade Neural Network Genetic Algorithm Space–Time Forecasting
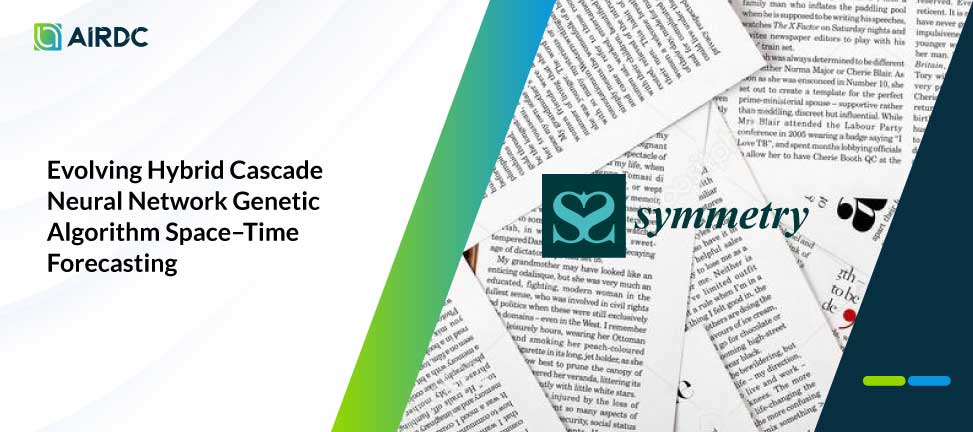
At the heart of time series forecasting, if nonlinear and nonstationary data are analyzed using traditional time series, the results will be biased. At the same time, if just using machine learning without any consideration given to input from traditional time series, not much information can be obtained from the results because the machine learning model is a black box. Purpose: In order to better study time series forecasting, we extend the combination of traditional time series and machine learning and propose a hybrid cascade neural network considering a metaheuristic optimization genetic algorithm in space–time forecasting. Finding: To further show the utility of the cascade neural network genetic algorithm, we use various scenarios for training and testing while also extending simulations by considering the activation functions SoftMax, radbas, logsig, and tribas on space–time forecasting of pollution data. During the simulation, we perform numerical metric evaluations using the root-mean-square error (RMSE), mean absolute error (MAE), and symmetric mean absolute percentage error (sMAPE) to demonstrate that our models provide high accuracy and speed up time-lapse computing.
Symmetry
Rezzy Eko Caraka, Hasbi Yasin, Rung Ching Chen, Noor Ell Goldameir, Budi Darmawan Supatmanto, Toni Toharudin, Mohammad Basyuni, Prana Ugiana Gio, Bens Pardamean