A Convolutional Neural Network-based Ancient Sundanese Character Classifier with Data Augmentation
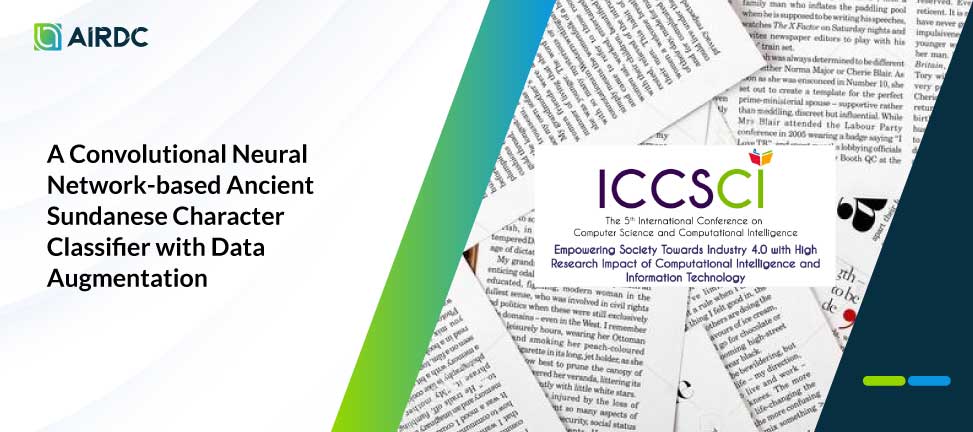
With an increasing interest in the digitization effort of ancient manuscripts, ancient character recognition becomes one of the most important areas in the automated document image analysis. In this regard, we propose a Convolutional Neural Network (CNN)-based classifier to recognize the ancient Sundanese characters obtained from a digital collection of Southeast Asian palm leaf manuscripts. In this work, we utilize two different preprocessing techniques for the dataset. The first technique involves the use of geometric transformations, noise background addition, and brightness adjustment to augment the imbalanced samples to be fed into the classifier. The second technique makes use of the Otsu’s threshold method to binarize the characters and only uses the usual geometric transformations for the data augmentation. The proposed network with different data augmentation processes is trained on the training set and tested on the testing set. Image binarization from the second technique can outperform the performance of the CNN-based classifier upon the first technique by achieving a testing accuracy of 97.74%.
International Conference on Computer Science and Computational Intelligence 2020
Alam Ahmad Hidayat, Kartika Purwandari, Tjeng Wawan Cenggoro, and Bens Pardamean