A Systematic Literature Review of Deep Learning Application in Multiclass Anomaly Detection for Chest Medical Imaging
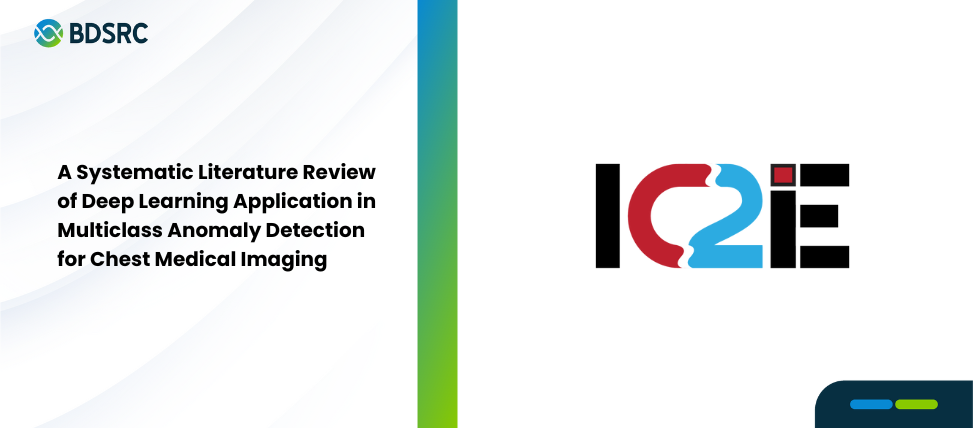
Medical imaging abnormality detection is challenging, but deep learning approaches have shown promise. This paper reviews the current state of the art in deep learning approaches for detecting abnormalities in chest medical imaging. To discover the trends, opportunities, and challenges associated with this field, 18 studies were selected from Google Scholar based on their titles, abstracts, and contents for extensive review to answer two research questions. The study found that the National Institutes of Health (NIH) Chest X-ray 14 dataset is the most used dataset for this task. Most research uses a single-modal approach, considering only image data as input, with X-ray being the more popular instrument. The transfer learning approach is leveraged by 8 out of 18 studies, with ResNet50 being the most popular network. MobileNetV2 has demonstrated competitive results compared to more robust networks. Preprocessing techniques such as image enhancement and data augmentation are leveraged by 61.1% of the reviewed studies and are shown to improve model performance.
Authors:
Philips, Bens Pardamean, Gregorius Natanael Elwirehardja, and Mahmud Isnan
6th International Conference on Computer and Informatics Engineering, IC2IE 2023