A Systematic Literature Review of CNN Approaches in Classifying Brain Tumor
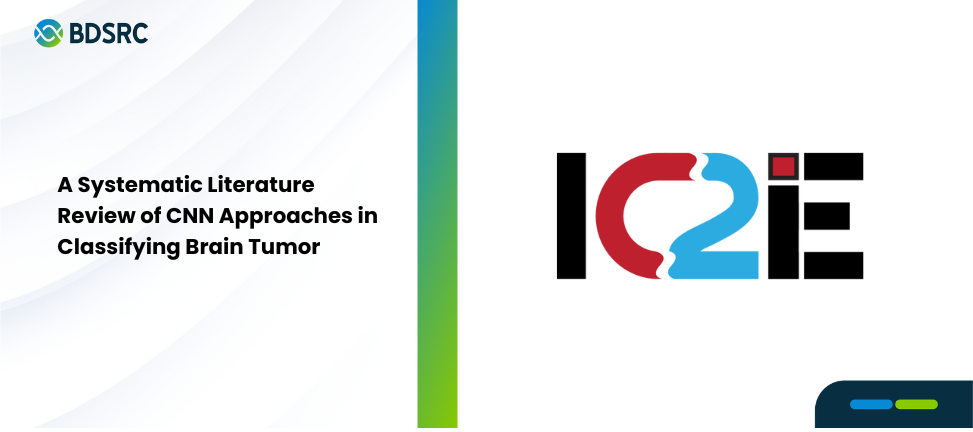
The ability of Convolutional Neural Networks (CNNs) to accurately discriminate between normal and tumorous brain tissues has been promising. The review focuses on the different CNN models, pre-processing methods, data augmentation, and Transfer Learning (TL) strategies used in this research. This Systematic Literature Review (SLR) collected the data from Google Scholar. The results of this study indicate that open-source datasets from Kaggle and Brain MRI Images for Brain Tumor Detection are the most used datasets. However, limited data and imbalanced class problems remain common challenges across various datasets. To overcome those challenges, using a larger dataset, oversampling, Generative Adversarial Network (GAN), federated learning, and Self-Supervised Learning (SSL) to handle the imbalance are the potential solution. Additionally, popular CNN architectures for brain tumor classification extensively use pre-trained models such as VGG16, VGG19, DenseNet121, DenseNet201, GoogleNet, ResNet-50, and Inception-v3. TL strategies are preferred, allowing CNNs to leverage knowledge from large datasets, improving generalization even with limited labeled data.
Authors:
Aedentrisa Yasmanda Paulindino, Bens Pardamean, and Gregorius Natanael Elwirehardja
6th International Conference on Computer and Informatics Engineering, IC2IE 2023