Detecting Nutrient Deficiency in Oil Palm Seedlings Using Multispectral UAV Images
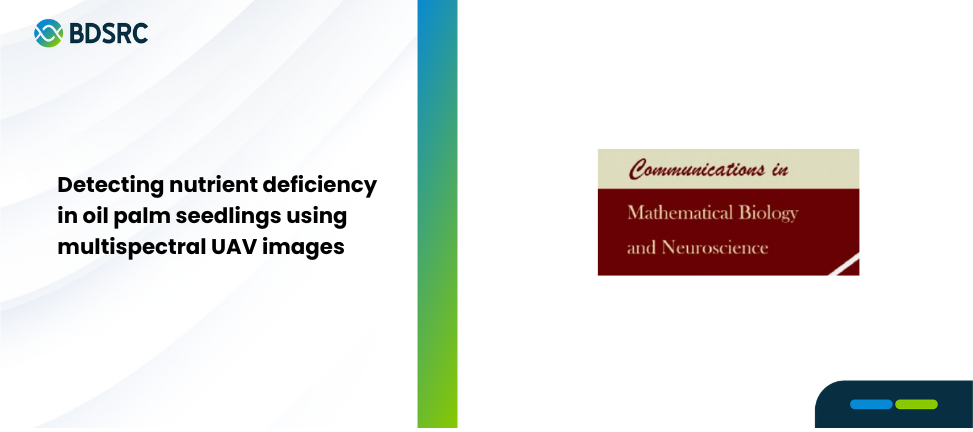
Remote sensing is driving transformation in the palm oil plantation industry with data acquisition and spatial analysis. Rapid identification of nutrients in oil palm plantations using a Remote Sensing approach provides benefits for plantation productivity and operations. The research was carried out at the PT Sampoerna Agro plantation, where 18-month-old oil palm seedlings received different intentional nutrient deficiency treatments. The research method used pixel-based classification algorithms with machine learning and Object-Based Image Analysis (OBIA) based segmentation to identify nutrient deficiencies in oil palm seedlings using multispectral Unmanned Aerial Vehicle (UAV) images. Both techniques were integrated with Random Forest and Support Vector Machine (SVM). The classification results using the OBIA-Random Forest classifier, reached an overall accuracy of 0.421, while the classification results using the OBIA-SVM classifier, reached an overall accuracy of 0.438. Meanwhile, by using the pixel-based supervised classification method, with the Random Forest and SVM algorithms, an overall accuracy of 0.413 and 0.438 were obtained. The overall accuracy of both classifiers was low, this is due to several nutrient deficiencies that could not be properly identified. However, nitrogen deficiencies could be identified accurately. Based on this research, the OBIA-SVM classifier has the best overall accuracy for identifying nutrient deficiencies in oil palm seedlings, with an overall accuracy of 0.438.
Authors:
Arief Ika Uktoro, Hermantoro -, Rengga Arnalis Renjani, Sandiaga Kusuma, Dwi Asmono, Ruli Wandri, Samsu Alam, Muchamad Nur Fanani Kramajaya, Angga Cahyo Riyanto, Teddy Suparyanto, Bens Pardamean
Communications in Mathematical Biology and Neuroscience (CMBN)