IMPROVING LUNG DISEASE DETECTION BY JOINT LEARNING WITH COVID-19 RADIOGRAPHY DATABASE
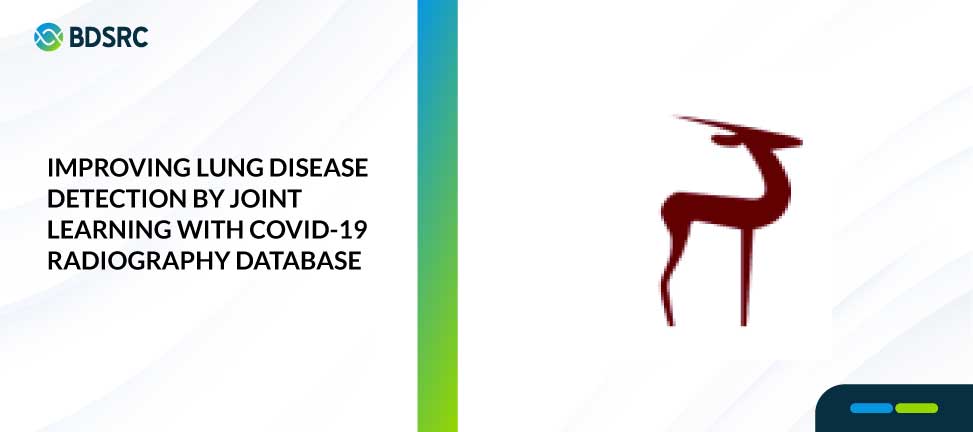
Diagnostic chest radiography is one of the most common imaging tests performed in medical practice. A radiology workflow goal is to detect, diagnose, and manage diseases using chest radiography in an automated, timely, and accurate manner. Radiography data have proved very effective for assessing COVID-19 patients, particularly for treating overcrowded emergency departments and hospitals. The use of Deep Learning (DL) methods in Artificial Intelligence (AI) has become dominant in detecting diseases via chest X-rays. This study utilized the COVID-19 Radiographic Database and the National Institutes of Health (NIH) Chest-Xray to study pre-training fine-tuning of the DL model on chest radiographic images. We investigate the robust network architecture in detail: DenseNet-121, in this dataset dual technique to improve insight into the different methods and their application to chest X-ray classification. Consequently, this dual dataset technique is able to provide better detection results for each cluster of lung diseases. AUC results obtained using DenseNet-121 reached an average of 82.16 percent, with the highest AUC reaching 99.99% in the cluster containing Viral Pneumonia lung disease.
Communications in Mathematical Biology and Neuroscience
Hery Harjono Muljo, Kartika Purwandari, Tjeng Wawan Cenggoro, Elwirehardja, Bens Pardamean