Phosphorylation Site Prediction Using Gradient Tree Boosting
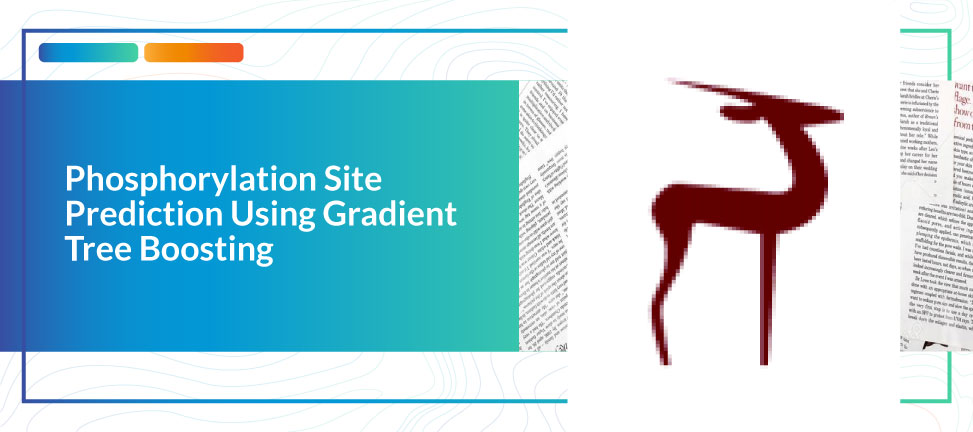
As one of the most important Post-Translational Modification (PTM), phosphorylation is responsible for cellular signaling pathways and activation of enzymes. With current computational power and algorithm, it is possible to process big data, especially biomedical data, to find a complicated pattern with reasonable computation time. Computational approach for phosphorylation site prediction is more time-efficient and need fewer resources compared to traditional. However, the accuracy of current computational methods for phosphorylation site prediction still needs to be improved. This paper aims to create a computational method for phosphorylation site prediction with better classification performance compared to previous studies. The data used in this research to train the XGBoost models are extracted features from 2 different databases from the previous studies. The test result show that our model gave the highest accuracy on 4 out of 6 datasets. To extend our research, the XGBoost model was retrained which focused on 100 most important features from previous experiment. However, the result does not imply that it has a better result compared to our first models. As the result showing that our models gave better accuracy compared to the previous studies in most of the datasets, we can conclude that XGBoost model is better in predicting phosphorylation sites compared to other methods.
Communications in Mathematical Biology and Neuroscience
Bharuno Mahesworo, Tjeng Wawan Cenggoro, Arif Budiarto, Favorisen Rosyking Lumbanraja, and Bens Pardamean