Evaluation Performance of SVR Genetic Algorithm and Hybrid PSO in Rainfall Forecasting
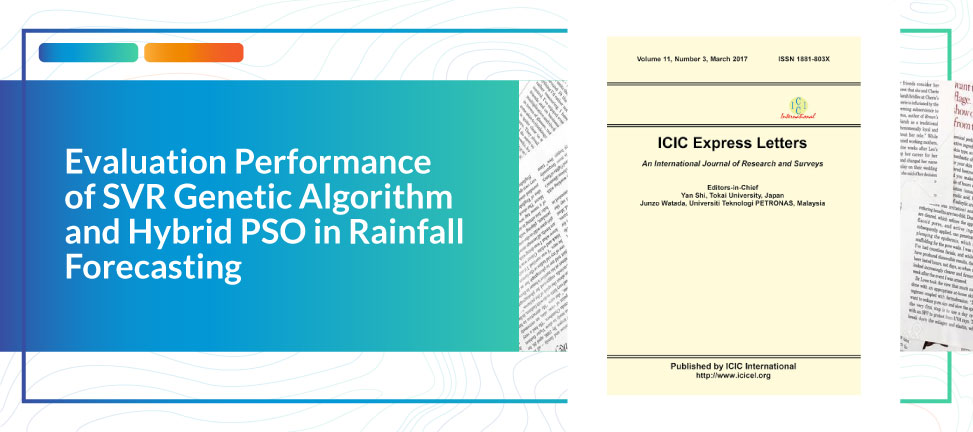
Climate is an essential natural factor which is dynamic and challenging to predict. The accurate climate prediction is needed. In this paper, we use support vector regression (SVR) with different kernels such as polynomial, sigmoid and RBF. At the same time, we employ genetic algorithm, particle swarm optimization, and hybrid particle swarm optimization. SVR-GA are population-based algorithms that allow for optimization of problems with the search space that is very broad and complex. This property too allows genetic algorithms to jump out of the local area optimum. In contrast with SVR-PSO and SVR-HPSO they do not have the genetic operation. In PSO only use internal velocity and have the memory which is essential to the algorithm. In this paper we compare SVR-PSO, SVR-HPSO and SVR-GA by comparing the input from the correlation and ARIMA in rainfall data. It was found that the input using correlation provides better accuracy than ARIMA.
ICIC Express Letters
Rezzy Eko Caraka, Rung Ching Chen, Toni Toharudin, Muhammad Tahmid, Bens Pardamean, and R. M. Putra