An End to End of Scalable Tree Boosting System
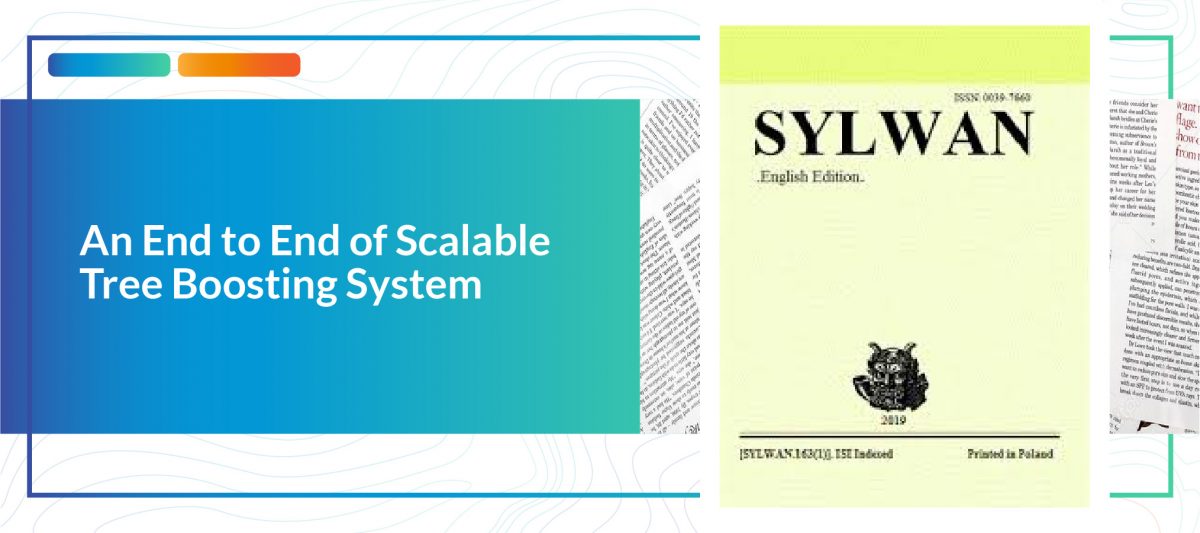
Feature selection in the health sector is essential to do. Moreover, an analysis of which variables are indeed important that affect specific diseases. In the 20th century, many machine learning methods gave the confidence to get good accuracy, one of which was the Scalable Tree Boosting System (XGBoost). This technique is the development of Random Forest, which is done by boosting to reduce each variable. In this paper, we will analyze the classification of diabetes mellitus. The results obtained that in the case studies 3 attributes confirmed important: `Two-hour postprandial blood sugar level`, `Fasting blood sugar level`, and ‘Triglyceride’ and reach the accuracy of 97.65%. XGBoost gives the best accuracy compared to Boruta, ID3, FFNN, SVM and Bayesian MCMC.
Sylwan 164(5)
Rung Ching Chen, Rezzy Eko Caraka, Arnita Piliang, Noor Ell Goldameir, Suwito Pomalingo, Andy Rachman, Toni Toharudin, Shao-Kuo Tai, Bens Pardamean